Improving defect identification in pipelines
28/09/2021This pilot in collaboration with Phillips 66 sought to explore how machine learning technologies could improve the identification of defects in energy pipelines to reduce the likelihood of leaks and impact on the environment.
The Challenge
In-line Inspection (ILI) tools, also known as smart pigs, are one of the primary inspection methods for evaluating pipeline integrity. These tools physically travel through pipes, scanning and measuring the pipeline’s wall thickness. A variety of data collection instruments are used by pigs including Magnetic Flux Leakage (MFL), Callipers and Ultrasound. Large quantities of data are analysed following the inspection to locate corrosion, dents, cracks and other pipeline features.
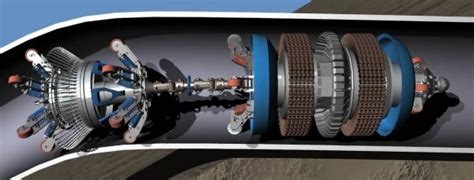
Currently there can be inconsistencies in the way pipeline features are identified. Non-threatening features may be exposed and sometimes features that might be a threat can fail to be targeted. Phillips 66 currently has systems in place, such as API 1163, to compensate for these uncertainties but sought new ways of interpreting the data collected from in-line inspections.
The Solution
UK based Conundrum AI provides an automated machine learning solution for industrial settings. The core technology allows analysis of sensor data for detecting, identifying and describing anomalies, along with highlighting inconsistencies in the data that might improve the quality of the overall results.
The Pilot
Phillips 66 provided over 11 million data points from previous surveys covering over 18km of pipelines. Some sections of piping had also been excavated to determine actual damage (truths) from internal corrosion, external corrosion and dents with metal loss.
After an initial data cleansing phase, Conundrum AI used the raw ILI data along with the truths to train their solution. One of the techniques used by Conundrum to achieve this was categorizing the features detected into buckets.
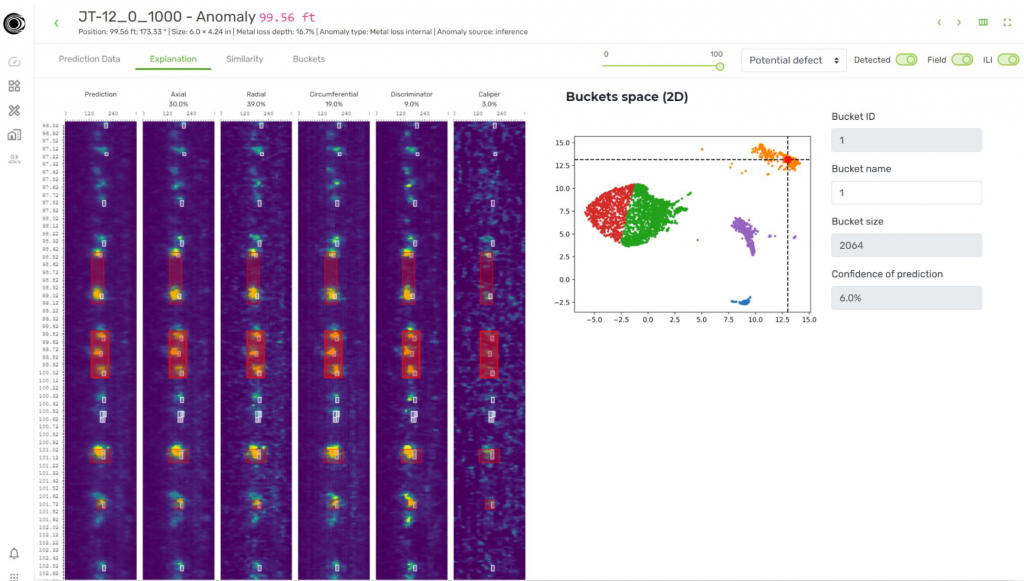
Following detection and classification, the severity of the defect was determined, e.g. degree of metal loss. The following charts compare the original ILI data interpretation with the newer machine learning results using Conundrum AI’s solution.
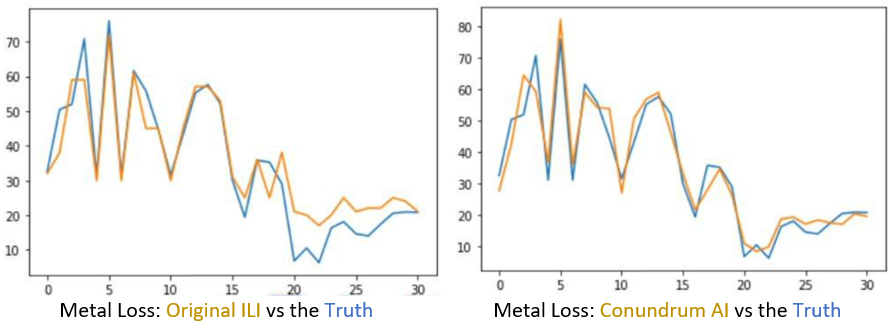
It is clear to see that Conundrum were able to train their solution to closely match the true features found from excavations.
The solution was designed to be deployed in the cloud allowing Phillips 66 to upload raw ILI data, perform analysis, update machine learning models and view results.
Results
Further ILI data was provided by Phillips 66 to test the capabilities of the new solution. Conundrum demonstrated how machine learning was able to assist in the interpretation of features
- Visual heatmaps show how the system made predictions
- Anomalies with similar raw data characteristics were grouped and presented as buckets
- New anomalies were identified
- Severity was expressed based on bucket, size and metal loss
- Confidence values were provided for predictions
“The Safetytech Accelerator program has given Phillips 66 the opportunity to work with Conundrum on an “outside-the-box” solution for identifying gaps that are inherent with pipeline in-line inspection tools. In-line inspection tools provide limited accuracy specifications, which along with inspection conditions and human error, can affect the overall performance of the system. Conundrum developed a software program that reviews pipeline inspection raw data and looks for signal discrepancies in similar reported anomalies. These discrepancies can be reviewed to see if there is a possible error with anomaly identification or classification and the pipeline operator can review if action is needed. Even with the challenging circumstances we’ve all faced over the last year, results have been promising and there is opportunity to refine and improve the analysis to a point of it becoming part of the overall integrity process.”
Joshua Bremner, Asset Integrity SME, Phillips 66
Industry-Wide Impact
In this pilot, Conundrum AI were able to accurately demonstrate quick analysis of ILI tool data. These results could give Engineers the capability to better identify misclassified pipeline features and potentially improve their prioritization of threats. The result is improved safety and impact on the environment across the industry.
About the Safetytech Accelerator
The Safetytech Accelerator is a non-profit established by Lloyd’s Register, bringing together corporates, startups and the wider technology community to collaborate and accelerate the adoption of technology in safety-critical industries.
In early 2020, the Accelerator was awarded the Global Lloyd’s List Award for Excellence in Data and Technology Innovation, in recognition of its excellence and impact in innovation, setting it apart from the competition worldwide. The industry-leading panel felt that the programme, the first of its kind globally, embodied everything that the Lloyd’s List Awards were established to champion, displaying important and impressive global impact for safety and risk in critical industries, including the maritime sector.
Read more about the initial challenge collaboration here.