Startup improves safety using deep learning-based computer vision.
13/11/2019The Challenge
Electrical cabinets are found globally, safely enclosing electrical wires and electronic equipment, preventing electrical shock hazards and protecting cabinet contents from the environment. Carrying out inspections and repairs on these cabinets poses many safety challenges, affecting workers, property and potentially environmental damage. Identifying anomalies of work done on these cabinets today can prove difficult, whether it’s missing fuse covers, mislabelled cable tags, or tools mistakenly left behind by the worker. The process infrastructure builders and operators use to detect and record these errors is quite manual and retrospective. A fitter uses their mobile device to submit photographs of work done on site that day to their case handler, who then reviews the images and flags any errors. Should an error be detected, such as an incomplete or incorrect job, the fitter is required to return to the electrical cabinet to rectify the issue and remove the hazard.
Scandinavian company Omexom (Infratek), a leader in building, operating and securing of critical infrastructure became a Safety Accelerator Challenge Partner in 2018, tapping into our network of best-in-class startup solutions, that could potentially speed up and automate the checking process. Omexom sought a digital solution to not only improve the speed of error detection on infrastructure assets, but to offer real-time detection and feedback to the worker, prior to leaving site, thus reducing corrective visits, repairs and safety hazards.
The Solution
The Accelerator team and programme partner Plug and Play collaborated closely with Omexom to define the scope of the challenge and to determine the relevant technology parameters. After a rigorous selection and judging process coordinated by the Safety Accelerator, Challenge Partners Omexom and a panel of expert industry judges, the three most promising startups pitched their ideas: Cape Town-based NumberBoost was the winner of this competitive process.
NumberBoost’s solution had been deployed successfully in other industries and during the pilot, they drew upon their experience and specific learnings from working with one of the largest mining companies in Africa.
With support from and in collaboration with LR, NumberBoost used their computer vision inspection technology powered by deep learning, to build a real-time error detection and feedback solution, to automatically detect anomalies in photos of work done on critical infrastructure, to ensure the job is done right the first time and to improve safety for the worker and third parties. The solution was deployed during a three-month pilot, funded by the Safety Accelerator.
“Getting the opportunity to be part of the Lloyd’s Register Safety Accelerator programme was an inspiring and very educational experience. The effectiveness of the implementation, from defining our challenge, to being presented in London with solutions from several highly competent startups was impressive. We chose to move forward with NumberBoost’s solution, based on their approach to the challenge, and their commitment to the task”.
“LR as facilitator meant that we got to meet companies that we in our own organization had not had the opportunity to get in touch with. Furthermore, the collaboration was easy, through web-based working meetings, before Alex from NumberBoost made the trip from South Africa to Norway, to fine-tune the pilot and present a working solution. LR was very accessible throughout, participating in presentations and testing the tool. I highly recommend taking part in the programme.” Stein-Olav Lervik, HSSEQ Director Norway, Omexom.
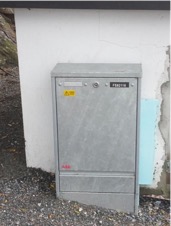
The Pilot
During the trial, the solution quickly detected errors and relayed the information to workers in real-time, prior to them leaving the site. An initial analysis of the Omexom historical job database showed electrical cabinets to be the most common asset worked on. This type of job was well suited for the pilot because it offered more data and more opportunity to improve efficiency.
Deep neural network models were trained on Omexom’s job-related photos to detect errors. Almost every possible combination of error type, photo angle and lighting condition was simulated, and several thousand photos were captured, to increase the overall dataset size that led to greater accuracy of the neural network model.
Seven common types of detected errors were the focus for this trial:
- Missing Fuse Covers
- Missing Snow Marker
- Missing Cable Tags
- Missing Cable Join Covers
- Missing Cable Insulation
- Tools Left Behind
- Trash Left Behind
A function of these machine learning models is that they return a level of confidence in their predictions with a human-in-the-loop approach used in cases where the models return low confidence, ensuring high overall system accuracy. Finally, the system includes a mechanism for the worker to provide corrective feedback to the model, in cases where outputs can be improved. The machine learning models are automatically re-trained as data is collected, so that the system’s accuracy improves over time as it is used.
Workers take before and after pictures of the job and real-time advice is provided, before the worker leaves the site.
The solution delivered powerful results, accurately detecting rubbish left behind at an 85% success rate, the absence of snow markers and cable insulation were detected with 80% accuracy, while detecting missing fuse covers was about 70% accurate.
“It was an absolute pleasure working with Omexom and the LR Safety Accelerator to apply our computer vision inspection technology to improving the safety of infrastructure inspections”. Alex Conway, CEO, NumberBoost. LR, Omexom and NumberBoost are collaborating on progressing the POC, exploring how the deep learning-based computer vision solution may be used to reduce errors across industries.
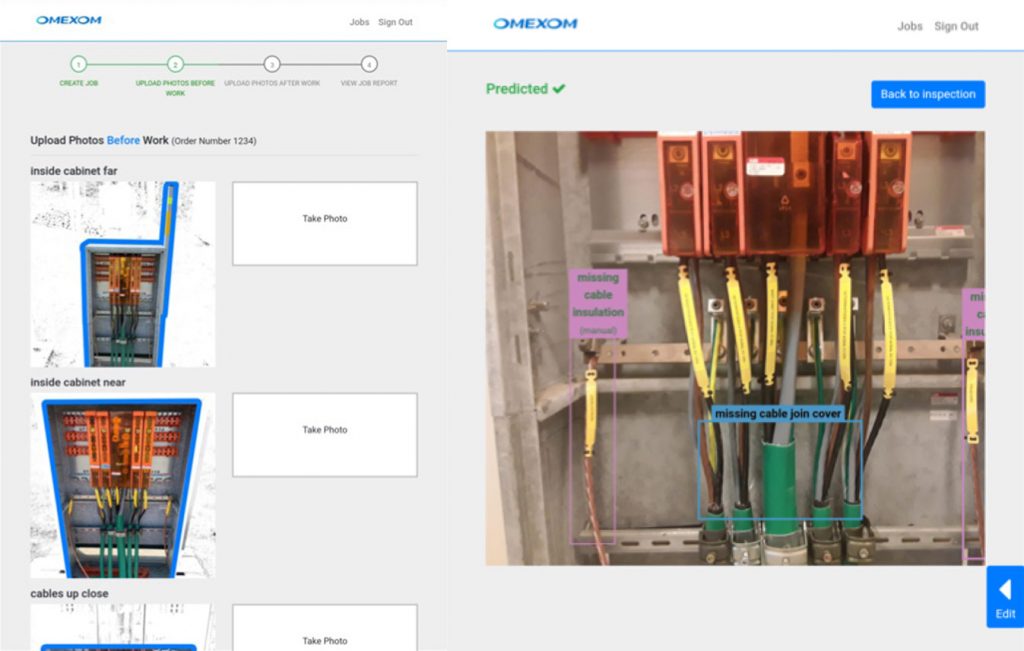
Industry-wide Impact
In this pilot we focused on electrical cabinets, but the same solution has the potential to detect and reduce unintentional human errors, improving safety and reducing the cost of maintenance across multiple industries that require engineers to visit remote sites. Repairs to machinery, pipelines and infrastructure could be validated before the engineer leaves the site, thus reducing time, cost and improving customer satisfaction.
About the Safetytech Accelerator
The Safetytech Accelerator is a dedicated open, not-profit initiative created by Lloyd’s Register Group and Lloyd’s Register Foundation, bringing together corporates, startups and the wider technology community to collaborate and accelerate the adoption of safetytech in safety-critical industries.
In early 2020, the Accelerator was awarded the Global Lloyd’s List Award for Excellence in Data and Technology Innovation, in recognition of its excellence and impact in innovation, setting it apart from the competition worldwide. The industry-leading panel felt that the programme, the first of its kind globally, embodied everything that the Lloyd’s List Awards were established to champion, displaying important and impressive global impact for safety and risk in critical industries, including the maritime sector.